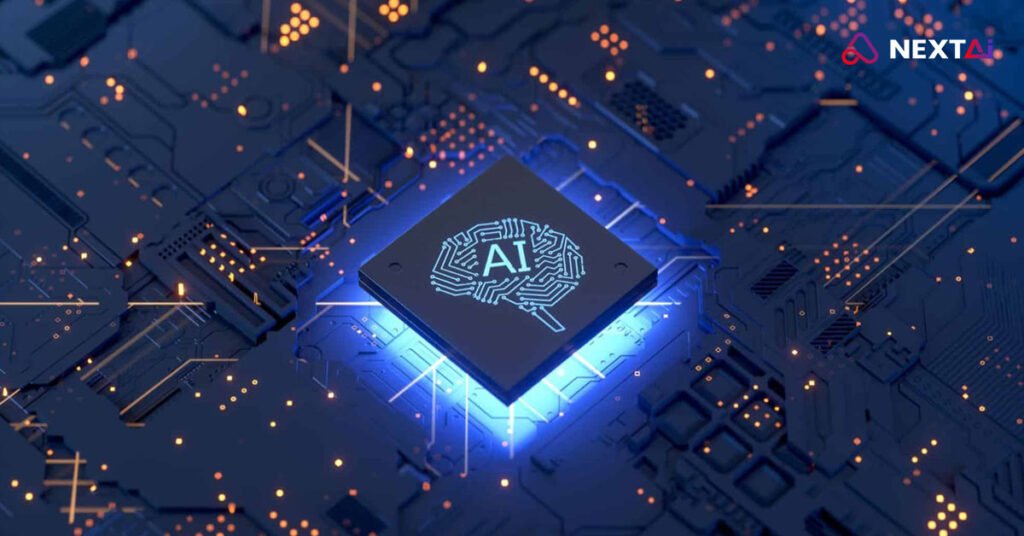
Leveraging AI in Data Engineering: Transforming Data Pipelines and Unlocking Insights
By Rajiv Rajkumar Bathija | AI in Data Engineering
As data grows exponentially in both volume and complexity, data engineering has become crucial for managing, processing, and delivering high-quality data. Artificial Intelligence (AI) is now playing a transformative role in data engineering, enhancing everything from data extraction to integration and quality assurance. By automating processes and providing intelligent insights, AI empowers data engineering teams to build more efficient, scalable pipelines and deliver valuable data-driven insights.
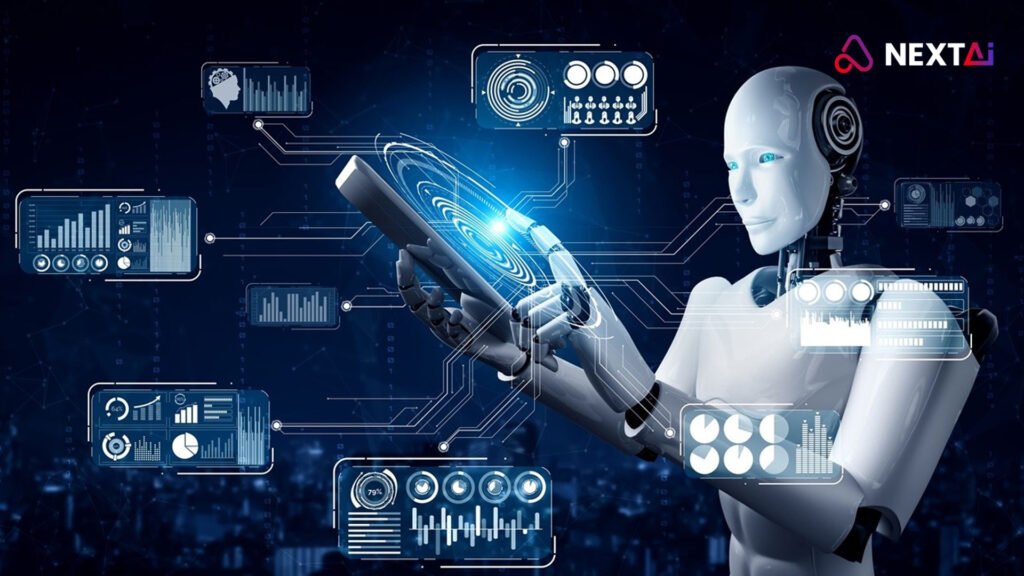
In this blog, I’ll explore the ways AI is revolutionizing data engineering, supporting tasks like data wrangling, pipeline optimization, data quality management, and more.
1. Automating Data Collection and Extraction
Data engineering starts with sourcing data from various systems, databases, and third-party sources. Traditional data collection processes are often manual, time-intensive, and prone to errors. AI can streamline this step through automated data extraction, using machine learning algorithms to parse, extract, and ingest data from structured and unstructured sources.
AI-powered tools can efficiently gather data from multiple sources, recognize patterns, and categorize data types, making data collection faster and more accurate. This not only reduces the time spent on initial data gathering but also improves the overall quality and consistency of the data.
2. Enhancing Data Wrangling and Transformation
Data wrangling, or transforming raw data into a structured format, is essential for data quality but can be labor-intensive. AI simplifies this process by automatically identifying patterns and relationships within datasets, transforming data into usable formats, and detecting anomalies.
For example, AI-driven tools can automatically clean, standardize, and normalize data, making it ready for analysis. This eliminates the need for manual data transformation and allows data engineering teams to focus on more complex tasks. With AI, teams can process larger datasets more efficiently, reducing time-to-insight and enabling faster decision-making.
3. Optimizing Data Pipelines for Efficiency and Scalability
Building and maintaining data pipelines is a core function of data engineering, but optimizing these pipelines can be challenging as data volume and variety increase. AI can help by analyzing pipeline performance and identifying bottlenecks, enabling continuous optimization for efficiency and scalability.
Machine learning models can be integrated into data pipelines to automate decision points, optimize data flows, and dynamically allocate resources. This results in pipelines that are more resilient and scalable, handling increased data loads without manual intervention. AI-driven pipeline optimization enhances both speed and reliability, ensuring that data processing remains smooth as datasets grow.
4. Improving Data Quality and Integrity
Data quality is critical in data engineering. Inconsistent, incomplete, or inaccurate data can lead to flawed insights and unreliable analytics. AI-powered quality assurance tools can automatically monitor data quality by identifying outliers, detecting duplicates, and validating data integrity in real time.
Through anomaly detection and data profiling, AI algorithms help data engineers catch errors early, ensuring high-quality data for downstream analytics. By automating these quality checks, AI reduces the risk of data issues going undetected, increasing confidence in data accuracy and reliability.
5. Supporting Data Integration and Unifying Diverse Data Sources
Data integration involves consolidating information from various systems and databases, often requiring complex transformations to achieve compatibility. AI assists by unifying data across sources, learning from previous integration efforts to recognize common data formats, mapping relationships, and merging data fields.
AI-powered data integration tools can automatically identify the best way to integrate disparate datasets, minimizing manual work and ensuring data consistency. This accelerates the integration process, allowing organizations to achieve a single, unified view of their data.
6. Enabling Predictive and Prescriptive Analytics
One of the most powerful applications of AI in data engineering is predictive and prescriptive analytics. By building models that analyze historical data, AI can forecast future trends, helping organizations anticipate outcomes and make proactive decisions.
Data engineers can incorporate these AI models directly into data pipelines, enabling real-time predictive analytics. For example, a predictive model could be integrated to provide demand forecasting, while a prescriptive model could suggest optimal resource allocations. AI-driven analytics empower organizations with actionable insights, enhancing data’s value and supporting informed, strategic decisions.
7. Simplifying Data Governance and Compliance
Data governance and compliance are essential to managing data privacy, security, and regulatory requirements. AI can help streamline these processes by monitoring data access, tracking data lineage, and flagging potential compliance issues in real time.
Through AI-driven compliance checks, data engineering teams can automate auditing processes, ensuring that data usage meets regulatory standards. This not only simplifies compliance management but also strengthens data governance, enhancing transparency and accountability in data handling.
8. Enabling Self-Healing Data Pipelines
In traditional data engineering workflows, when an error or failure occurs in a data pipeline, engineers must often intervene manually to troubleshoot and resolve the issue. AI enables “self-healing” capabilities in data pipelines by identifying and correcting issues automatically. When a pipeline encounters an unexpected problem, AI can diagnose the cause, apply fixes, and restore functionality with minimal human intervention.
Self-healing pipelines minimize downtime, improve resilience, and reduce the time data engineers spend on troubleshooting. This functionality is especially valuable in dynamic, real-time data environments where disruptions can significantly impact operations.
9. Enhancing Collaboration with Natural Language Processing (NLP)
Natural Language Processing (NLP) helps bridge the gap between data engineering teams and business stakeholders by simplifying communication and data queries. AI-driven NLP tools allow users to interact with data through simple language queries, making data insights more accessible.
For example, business users can ask questions about data trends or patterns, and NLP-powered AI can generate reports, visualizations, or even written summaries. This democratizes access to data and enhances collaboration, allowing non-technical team members to leverage data without needing in-depth engineering expertise.
Final Thoughts: The Future of Data Engineering with AI
AI is revolutionizing data engineering by streamlining workflows, enhancing data quality, and enabling predictive insights that drive decision-making. From automating data transformation to supporting compliance, AI tools empower data engineering teams to work faster, smarter, and more accurately, handling complex data challenges with ease. As AI technology continues to advance, it will further elevate data engineering, helping organizations unlock the full potential of their data.
If you’re interested in exploring how AI can transform your data engineering processes, let’s connect and discuss AI-powered solutions that can optimize your workflows, improve data quality, and provide actionable insights.
Author: Rajiv Rajkumar Bathija
#DataEngineering #ArtificialIntelligence #AIAutomation #DataPipelines #PredictiveAnalytics #RajivRajkumarBathija #DataQuality #AIinDataEngineering