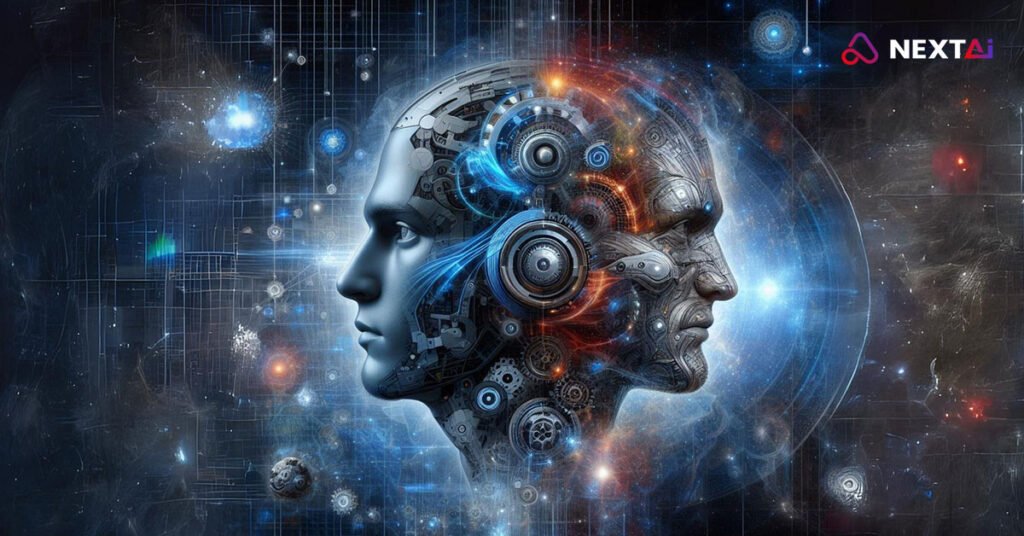
AI and Data Engineering: How Intelligent Automation is Redefining Data Workflows
By Rajiv Rajkumar Bathija | AI and Data Engineering
At 60, with over 35 years of experience in the tech industry, I’ve witnessed the evolution of data engineering from manual data management to today’s AI-powered workflows. As a thought leader and speaker in the AI community, I’m passionate about helping organizations harness Artificial Intelligence (AI) to drive efficiency, streamline workflows, and uncover valuable insights. In this article, I’ll share how AI-driven automation is reshaping data engineering, transforming complex data processes, and empowering businesses to make faster, more informed decisions.
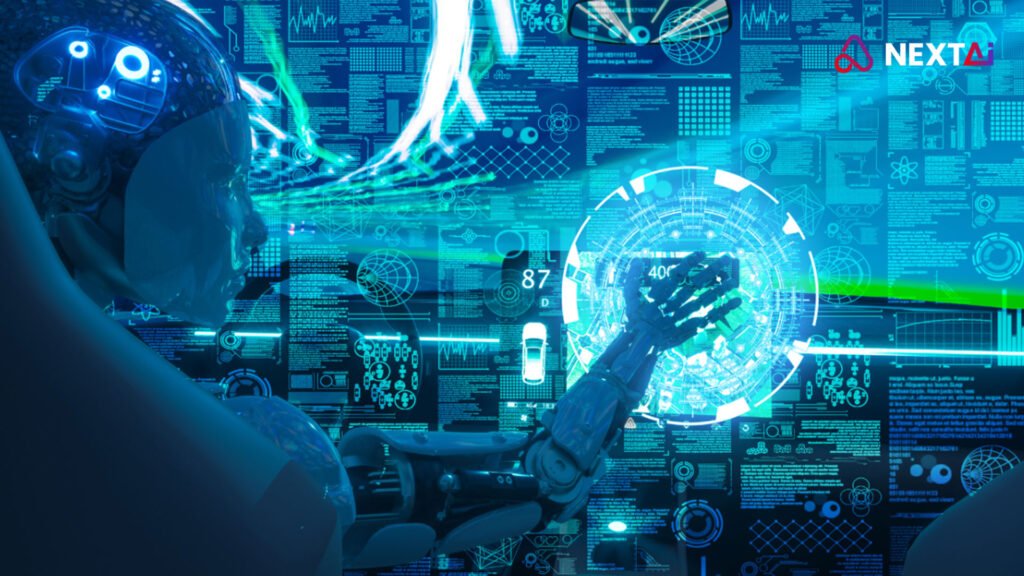
Data engineering involves a series of complex, resource-intensive tasks, from data collection and integration to quality assurance and processing. As data volumes expand, traditional data engineering methods can fall short in efficiency, scalability, and speed. AI-driven automation offers a solution, allowing data engineers to optimize workflows, improve data quality, and reduce manual effort. Here’s how intelligent automation is revolutionizing data engineering workflows and reshaping the future of data management.
1. Automating Data Ingestion for Faster Access
Data ingestion—the process of gathering and importing data from multiple sources—is foundational in data engineering but can be time-consuming. AI-driven automation enables continuous data ingestion by identifying, extracting, and integrating data from various sources in real time. This approach speeds up the data collection process and ensures that organizations have immediate access to up-to-date information.
Automated data ingestion also improves data consistency, making it easier for data engineers to standardize and consolidate information from multiple sources. This streamlined process enables faster data access, giving teams the agility needed to respond to market changes and business needs promptly.
2. Transforming Data Cleansing with AI
Data quality is critical in data engineering, but data cleansing—removing inaccuracies, duplicates, and inconsistencies—can be labor-intensive. AI simplifies this by automating data cleansing tasks, using machine learning algorithms to detect and correct errors, standardize values, and remove irrelevant data points.
For instance, AI can identify duplicate entries or detect patterns that indicate incorrect data. With AI-driven data cleansing, data engineers can maintain high-quality datasets without spending countless hours on manual checks. This automated process not only enhances accuracy but also accelerates data preparation, allowing teams to analyze data faster.
3. Intelligent Data Transformation for Greater Flexibility
Data transformation is essential for making raw data compatible with analytics platforms, but traditional methods are often rigid and complex. AI-driven automation introduces flexibility into data transformation by automatically identifying optimal formats, structures, and types for different data applications.
Using machine learning models, AI can analyze datasets, suggest transformations, and even apply custom formatting. This level of intelligent data transformation allows businesses to adapt quickly to new data sources and formats, enabling seamless integration with analytics tools and applications.
4. Boosting Data Quality Management with Automated Validation
Maintaining data quality is an ongoing process, and AI is essential for managing this efficiently. AI-powered validation tools can continuously monitor data for anomalies, inconsistencies, and errors, ensuring that quality is maintained throughout the data pipeline.
For instance, AI algorithms can automatically detect missing values, incorrect formats, or unexpected outliers, flagging them for review. Automated data quality management not only reduces the risk of errors in analytics but also improves confidence in data-driven decisions, enhancing the overall reliability of business intelligence efforts.
5. Optimizing ETL Workflows with AI-Driven Efficiency
Extract, Transform, Load (ETL) workflows are central to data engineering, but managing them manually can be cumbersome and resource-heavy. AI-driven automation optimizes ETL workflows by streamlining each step, from data extraction to loading, with minimal human intervention.
For example, AI can automate data extraction from multiple sources, dynamically adjust transformation rules based on data patterns, and load the transformed data into the target storage system. By automating these ETL tasks, data engineering teams can process larger data volumes at higher speeds, supporting more complex analytics initiatives without compromising on accuracy or efficiency.
6. Enhancing Data Security with AI-Powered Monitoring
Data security is critical in any data engineering process, and AI enhances security by continuously monitoring data activity for suspicious behavior. Machine learning algorithms can detect anomalies that may indicate unauthorized access, data tampering, or potential breaches, allowing organizations to respond promptly.
Automated AI-driven monitoring tools track user access patterns, flag unusual data access requests, and alert teams to potential risks in real time. This proactive approach to security helps organizations protect sensitive information, minimize data risks, and comply with regulatory standards.
7. AI-Driven Data Governance for Compliance and Transparency
Data governance is essential for managing data access, security, and compliance, particularly as organizations face increasing regulatory scrutiny. AI supports data governance by automating compliance checks, tracking data lineage, and enforcing access controls.
With AI-driven automation, data engineers can maintain detailed records of data usage, access permissions, and changes, ensuring that data remains secure and compliant with standards like GDPR and CCPA. Automated data governance enables businesses to achieve transparency and accountability, reducing the risk of non-compliance and building trust with customers and stakeholders.
8. Enabling Self-Optimizing Pipelines with AI
One of the most exciting developments in AI-driven data engineering is the concept of self-optimizing data pipelines. By using machine learning algorithms, AI can continuously monitor pipeline performance, identify bottlenecks, and make real-time adjustments to improve efficiency.
For instance, if a particular step in the data pipeline experiences a delay, AI can reallocate resources or adjust workflows to maintain smooth data flow. These self-optimizing pipelines reduce downtime, improve scalability, and ensure that data processing remains efficient even as data volumes grow. This dynamic adaptability is key to maintaining resilient, high-performance data pipelines.
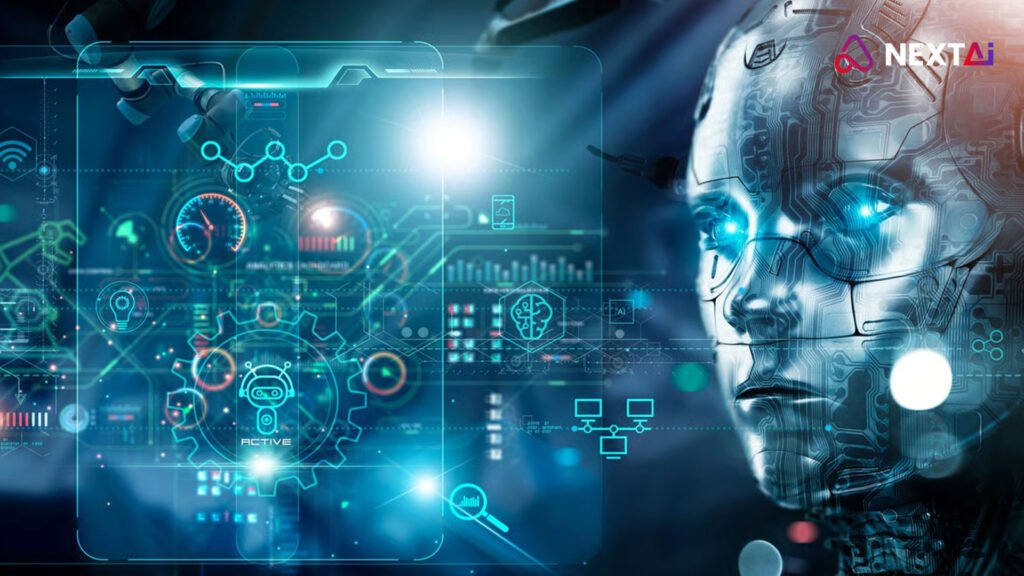
Final Thoughts: The Future of Data Engineering with AI-Driven Automation
AI-driven automation is redefining data engineering workflows, enabling teams to handle larger datasets, improve data quality, and ensure security and compliance—all while reducing manual effort. With my decades of experience in the industry, I’ve seen how AI has transformed data workflows, making data engineering not only faster and more efficient but also more adaptive and intelligent.
As a senior AI expert, I am excited about the future of data engineering and the potential of AI-driven solutions to unlock new levels of productivity and innovation. For organizations ready to embrace the future of data engineering, intelligent automation offers a powerful foundation to drive growth, enhance agility, and achieve sustainable success.
If you’re interested in exploring how AI can transform your data engineering processes and support your business goals, let’s connect. Together, we can harness the power of AI to build smarter, faster, and more resilient data workflows for a data-driven world.
#DataEngineering #ArtificialIntelligence #AIAutomation #RajivRajkumarBathija #DataPipelines #DataQuality #ETLAutomation #DataGovernance
Author: Rajiv Rajkumar Bathija